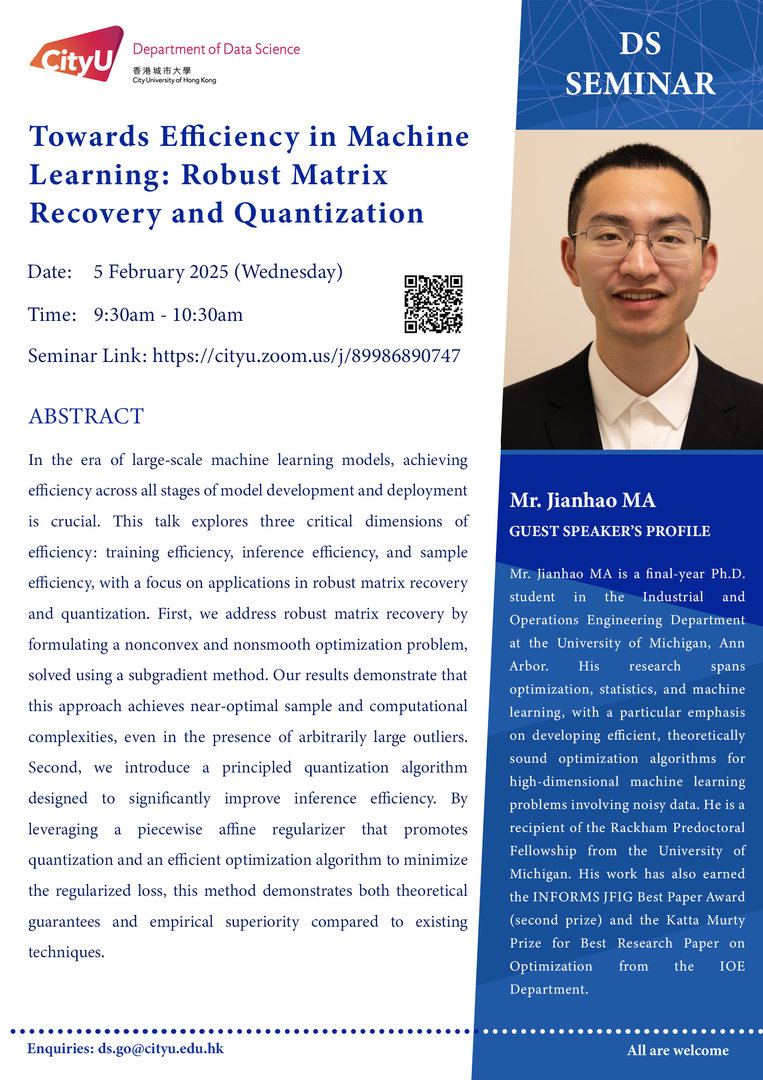
In the era of large-scale machine learning models, achieving efficiency across all stages of model development and deployment is crucial. This talk explores three critical dimensions of efficiency: training efficiency, inference efficiency, and sample efficiency, with a focus on applications in robust matrix recovery and quantization. First, we address robust matrix recovery by formulating a nonconvex and nonsmooth optimization problem, solved using a subgradient method. Our results demonstrate that this approach achieves near-optimal sample and computational complexities, even in the presence of arbitrarily large outliers. Second, we introduce a principled quantization algorithm designed to significantly improve inference efficiency. By leveraging a piecewise affine regularizer that promotes quantization and an efficient optimization algorithm to minimize the regularized loss, this method demonstrates both theoretical guarantees and empirical superiority compared to existing techniques.
Speaker: Mr. Jianhao MA
Date: 5 February 2025 (Wednesday)
Time: 9:30am – 10:30am
Zoom: Link
Poster: Click here
Latest Seminar
Biography
Mr. Jianhao MA is a final-year Ph.D. student in the Industrial and Operations Engineering Department at the University of Michigan, Ann Arbor. His research spans optimization, statistics, and machine learning, with a particular emphasis on developing efficient, theoretically sound optimization algorithms for high-dimensional machine learning problems involving noisy data. He is a recipient of the Rackham Predoctoral Fellowship from the University of Michigan. His work has also earned the INFORMS JFIG Best Paper Award (second prize) and the Katta Murty Prize for Best Research Paper on Optimization from the IOE Department.